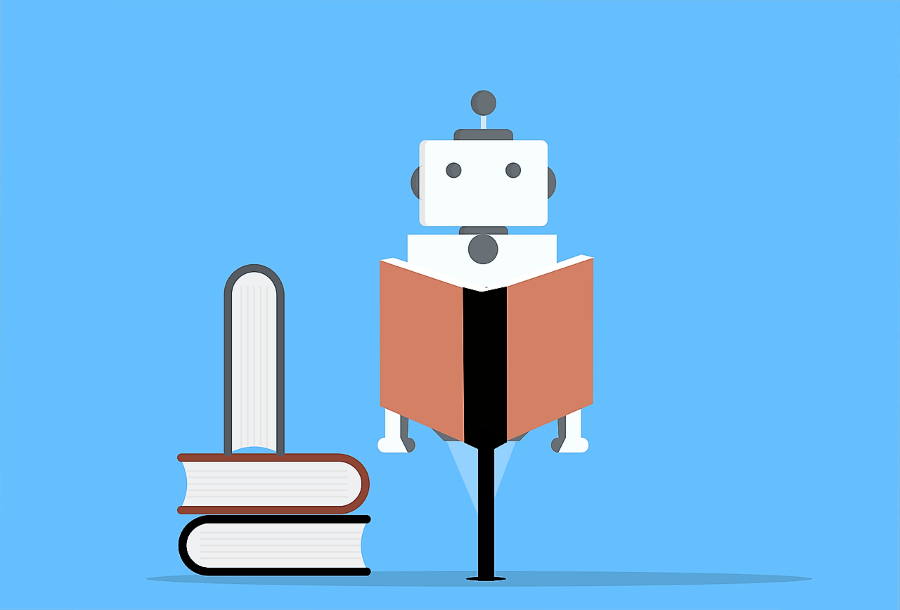
Scientists at the S N Bose Centre for Basic Sciences (SNBNCBS), an autonomous institute of the Department of Science and Technology (DST), Govt. of India, have used Machine learning (ML) to help predict new materials for nano alloys, semiconductors & rare earths.
Technically speaking, SNBNCBS scientists used ML to develop a design map of alloys at the nano-scale which can help predict the match of pairs of metals that can form bimetallic nanoalloys (composed of two different metal elements).
Fir uninitiated, Rare Earths are a group of 15 elements in the periodic table and a substantial part of these elements are less common and more expensive. Rare earth compounds with permanent magnetic properties are used in loudspeakers and computer hard drives. Of these, 17 elements of the periodic table like Neodymium, Lanthanum and so on are found sparsely on the earth’s crust, and their supply is monopolized by the countries where their mines happen to be located.
S.N Bose Centre has used machine learning to search for cheaper substitutes of naturally occurring rare earth material. By painstakingly creating a database of rare earth compounds and their attributes and then constructing a machine-learning model, SNBNCBS researchers have predicted a list of potential candidates for permanent magnets whose cost will be less than $100 per Kg.
![]() |
Machine learning model for core-shell bimetallic nanoclusters |
SNBNCBS team successfully connected Machine Learning with nanoscience in tracing the mixing patterns of metal atoms in nanoclusters and formed a basis for the design map, which can help select the pairs of metals for nanocluster alloys. This design map developed by the scientists will be tested out in the nano laboratories at the Moscow State University and also at the S.N Bose Centre.
Besides, in an another study by SNBNCBS team, which is on the heterogeneous structure formed at the junction of two dissimilar semiconductors, they have established that using machine learning, hetero-structure types used in hetero-junctions of two semiconductors which are at the heart of devices like LEDs, solar cells and photovoltaic devices, can be predicted fairly accurately.
The ML model designed by the S.N Bose team predicted 872 unknown semiconductor hetero-structures of type 2 where the electrons and holes align themselves in A semiconductor and B semiconductor, respectively, giving rise to a desirable hetero-structure for semiconductor gadgets.
In their paper published in the Journal of Physical Chemistry, they investigated the key attributes driving the core−shell morphology using the statistical tool of machine learning applied on this large data set. Core-shell structures with lighter metals having lower atomic numbers in the core were classified as Type 1, and those having the heavier metals in the core were classified as Type 2. A number of attributes were built to characterise each data point in the set. The performance of the ML model was tallied with existing experimental data, and the ML model was proved to be reliable.